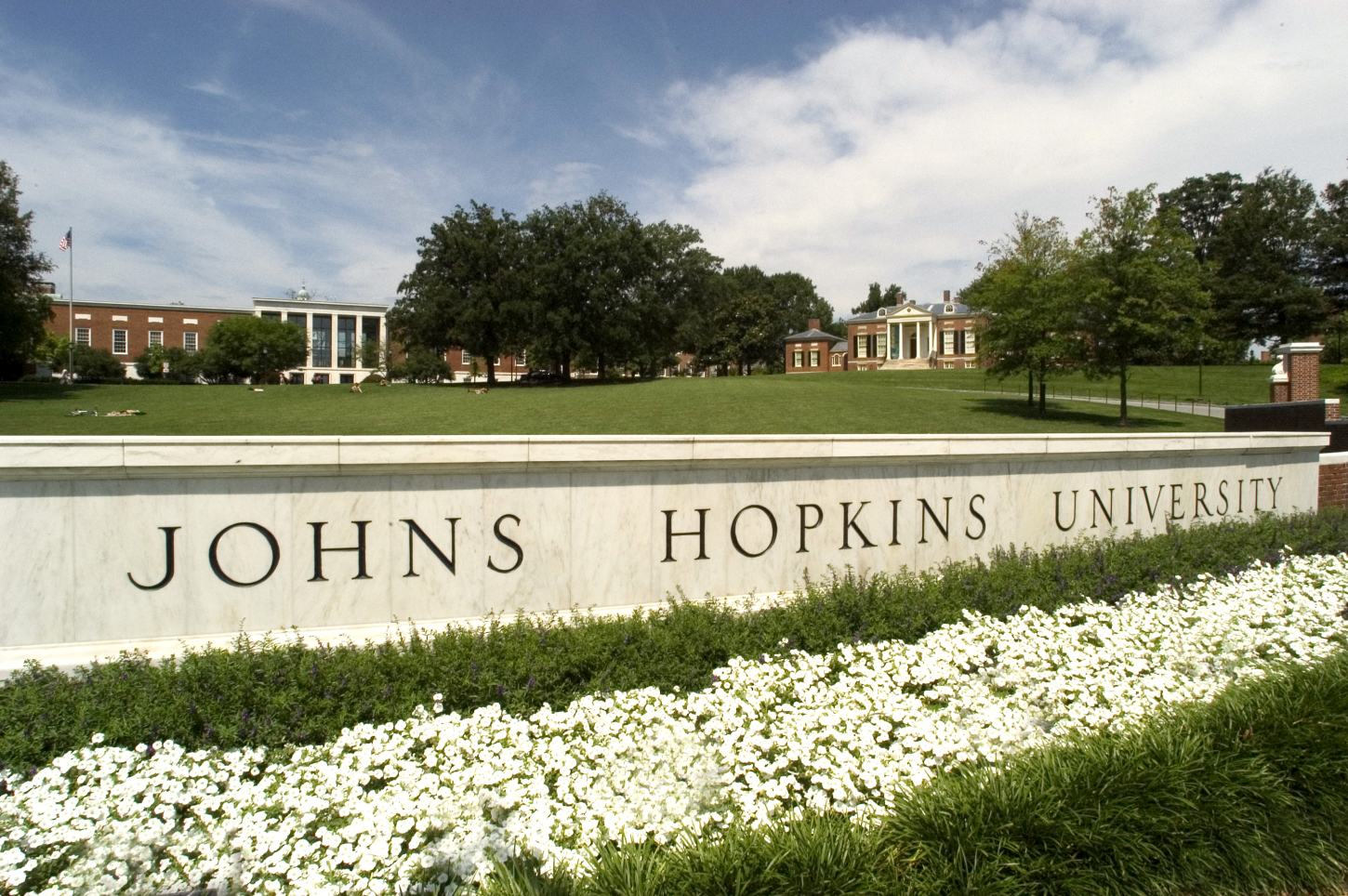
580.691/491 Learning, Estimation, and Control
The course introduces modern techniques in mathematical analysis of biomedical data. Techniques include maximum likelihood, estimation theory via Kalman equation, application of Kalman to Brain-Machine Interfaces, state-space models, Bayesian estimation, classification of labeled data, dimensionality reduction, clustering, expectation maximization, and dynamic programming and reinforcement learning via the Bellman equation.
- Introduction
- Perceptron.
- Homework: predicting which movie to suggest data
- Extra credit: handwritten digit classification
- Homework: predicting which movie to suggest data
- Perceptron.
- Regression and maximum likelihood
- Probability theory. Bayes rule, expected value and variance of random variables and sum of random variables, expected value of random variables raised to a power, Binomial distribution, Normal distribution
- Lecture notes
- Homework: probability theory
- Lecture notes
- Least mean squared loss function, batch learning and the normal equation, cross validation.
- Lecture notes
- Homework: classify using regression. Data set.
- Newton-Raphson, LMS and steepest descent. Newton-Raphson, LMS and steepest descent with Newton-Raphson, weighted least-squares, regression with basis functions, estimating the loss function for learning in humans.
- Lecture notes
- Homework: moving centers of Gaussian bases.
- Lecture notes
- Backpropagation.
- Examples of estimation and learning in biological systems (chapter 4.1-4.5)
- Maximum likelihood. Maximum likelihood estimation; likelihood of data given a distribution; ML estimate of model weights and model noise, integration of multiple sensory data.
- Probability theory. Bayes rule, expected value and variance of random variables and sum of random variables, expected value of random variables raised to a power, Binomial distribution, Normal distribution
- Estimation theory
- State estimation of dynamical systems (chapter 4.6 and 4.7). Optimal parameter estimation, parameter uncertainty, state noise and measurement noise, adjusting learning rates to minimize model uncertainty. Derivation of the Kalman filter algorithm.
- Kalman filter and signal dependent noise (chapter 4.9 and 4.10).
- State estimation of dynamical systems (chapter 4.6 and 4.7). Optimal parameter estimation, parameter uncertainty, state noise and measurement noise, adjusting learning rates to minimize model uncertainty. Derivation of the Kalman filter algorithm.
- Bayesian estimation
- Applying Kalman to Brain Machine Interface. Bayes estimation and its relationship to Kalman filters (chapter 5.1). Factorization of joint distribution of Gaussian variables.
- Homework: posterior distribution with two observed data points; maximizing the posterior directly.
- Causal inference (chapter 5.2 and 5.3). The problem of deciding between two generative models.
- Examples from biological learning: Kamin blocking, backward blocking, and comparisons between Kalman learning and LMS.
- MAP estimation.
- Applying Kalman to Brain Machine Interface. Bayes estimation and its relationship to Kalman filters (chapter 5.1). Factorization of joint distribution of Gaussian variables.
- Classification with labeled data
- Fisher linear discriminant, posterior probabilities. Classification using posterior probabilities with explicit models of densities, Kernel estimates of density.
- Lecture notes
- Homework
- Lecture notes
- Extra credit Homework
- Lecture notes
- Lecture notes
- Logistic regression. Iterative re-weighted least squares estimation
- Fisher linear discriminant, posterior probabilities. Classification using posterior probabilities with explicit models of densities, Kernel estimates of density.
- Dimensionality reduction and clustering
- Dimensionality reduction: Principal component analysis
- Homework: spike sorting data
- Homework: spike sorting data
- Dimensionality reduction: t-SNE
- Unsupervised classification: Mixture models, K-means algorithm, and Expectation-Maximization.
- Homework: image segmentation. Imagedata
- Homework: image segmentation. Imagedata
- EM and maximizing the complete log likelihood.
- Dimensionality reduction: Principal component analysis
- Bellman equation and optimal control
- Lagrange multipliers and constraint minimization (chapter 10)
- Open loop control with signal-dependent noise. (chapter 11)
- Introduction to optimal feedback control (chapter 12.1-12.3). Bellman equation.
- Optimal feedback control of linear dynamical systems with non-signal dependent noise.
- Optimal feedback control with signal dependent noise
- Homework: Mountain car problem via Bellman equations
- Homework: Mountain car problem via Bellman equations
- Reinforcement learning
- Lagrange multipliers and constraint minimization (chapter 10)